Kim H. Esbensena and Francis F. Pitardb
aConsultant, Copenhagen Ø, Denmark, www.kheconsult.com. Adjunct professor, Aalborg University (AAU), Denmark; Adjunct professor, Geological Survey of Denmark and Greenland (GEUS); Professeur associé, Université du Québec à Chicoutimi (UQAC), Québec; Guest professor University of Southeast Norway (USN); Guest professor (2018) Recinto Universitario de Mayaguez, Puerto Rico
bFrancis Pitard Sampling Consultants, LLC, 14800 Tejon Street, Broomfield, Colorado, 80023, USA
DOI: https://doi.org/10.1255/sew.2022.a22
© 2022 The Authors
Published under a Creative Commons BY licence
Experience from the realm of scientific conferences around the world reveals that many authors and presenters are unconsciously often shifting between the term error and the term uncertainty without a clear vision of what is the subtle difference between them. The same applies to a large swath of the scientific literature, in which these two concepts are often used synonymously—a scientific flaw of the first order. There is always a difference, regardless of how small, signifying a non-zero difference between the true, unknown content aL of a lot L and the estimated (analysed) content of a sample hereof, aS. Is this difference an error or an uncertainty? Many practitioners will call it an error. This estimate of the lot concentration per force has to be made based on the minute (hopefully representative) analytical aliquot. As is well-known within the sampling community it is all about how to be able to secure a documentable representative analytical sample “from-lot-to-aliquot” upon which to carry out valid analysis.
Introduction and background
Tradition in many scientific fields has established the word “error” as common practice, though for some this term implies a mistake, an error that could or should have been prevented. This possible accountability does not sit well with some statisticians, which prefer the word “uncertainty” instead, because this implies no culpability. This has given rise to the need for a clear distinction between “error” and “uncertainty”, which is made all the more pressing since these two terms are often used inter alia in less strict usages; and it certainly does not help that what is known in certain European statistical circles as errors, are denoted uncertainty in certain American communities. Of course, everybody claims to be right ….
See Reference 1 for an almost random example of the complete confusion that is out there. So “uncertainty … is measured by the amount of error” – the confusion is complete!
Because of this state of affairs, the Council of the International Pierre Gy Sampling Association’s Advisory Group has decided to clean up at least our own act—thus this “Sampling Column”.
The TOS vs statistics
In the Theory of Sampling (TOS) realm it has been overwhelmingly demonstrated that there are both sampling errors and sampling uncertainties. The effects of some sampling errors can be preventatively minimised, or even eliminated (ISE), while some sampling uncertainty for a given sampling protocol is inevitable. The job is to minimise this remainder (CSE). Gy2 stated: “With the exception of homogeneous materials, which only exist in theory, the sampling of particulate materials is always an aleatory (Aleatory: depending on the throw of a dice or on chance; random.) operation.” There is always an uncertainty, regardless of how small it is, between the true, unknown content aL of the lot L and the true, unknown content aS of the sample S.
Thus, because the word “uncertainty” is not well suited in the real-world context of heterogeneous materials and lots, the term “error” was decided upon for the TOS,2 making it clear that this does not necessarily imply culpability—but it may. The sampling errors which are subject to the possibility of elimination shall and must be so (ISE)! If not, someone or somebody is in effect responsible for committing an error resulting in a sampling bias (see below), which unavoidably results in unnecessary inflation of MUtotal—thus increasing the total sampling uncertainty. This most definitely constitutes an error for which someone is responsible (it could be because of faulty or inferior equipment, because of an inferior standard or ditto procedural description—or because of an incompetent sampler or a ditto supervisor). The essence of the stand described here has been delineated forcefully by Pitard3 (p. 33) who graciously informs the reader that this stand originates with Pierre Gy,2 see also Reference 4.
Gy’s choice was especially justified for an Increment Delimitation Error (IDE), Increment Extraction Error (IEE), Increment Weighting Error (IWE) and Increment Preparation Error (IPE). Because the magnitude of these errors is dictated by the ignorance, unwillingness or negligence of operators, managers and manufacturers to make these errors negligible by following the rules of Sampling Correctness stipulated in the TOS. For these errors, the word uncertainty would be totally inappropriate. Therefore, in any project, if management is due diligent, the word error should not exist and only uncertainties remain; the problem is we are living in a world very far from perfect where the TOS is not yet mandatory knowledge for everyone in the business of creating an important analytical database. A few examples may clarify the validity of our approach in the Theory of Sampling realm.
Fact box: Too many conflicting definitions
“Uncertainty refers to epistemic situations involving imperfect or unknown information. It applies to predictions of future events, to physical measurements that are already made, or to the unknown. Uncertainty arises in partially observable or stochastic environments, as well as due to ignorance, indolence, or both”. Wikipedia, https://en.wikipedia.org/wiki/Uncertainty
“Uncertainty of a measured value is an interval around that value such that any repetition of the measurement will produce a new result that lies within this interval”. B. Accuracy vs. Precision, and Error vs. Uncertainty. https://www.bellevuecollege.edu/physics/resources/measure-sigfigsintro/b-acc-prec-unc/
Authors comment: a definition only involving measurement uncertainty
Defining Error and Uncertainty
“Some of the terms in this module are used by different authors in different ways. As a result, the use of some terms here might conflict with other published uses. The definitions used in this module are intended to match the usage in documents such as the NIST Reference on Constants, Units and Uncertainty.
For example, the term error, as used here, means the difference between a measured value and the true value for a measurement. Since the exact or ‘true’ measured value of quantity can often not be determined, the error in a measurement can rarely be determined. Instead, it is more consistent with the NIST methods to quantify the uncertainty of a measurement.
Uncertainty as used here means the range of possible values within which the true value of the measurement lies. This definition changes the usage of some other commonly used terms. For example, the term accuracy is often used to mean the difference between a measured result and the actual or true value. Since the true value of a measurement is usually not known, the accuracy of a measurement is usually not known either.”
Peter Bohacek and Greg Schmidt, What is Measurement and Uncertainty?. https://serc.carleton.edu/sp/library/uncertainty/what.html [accessed 22 August 2022]
Case #1: A necessary sample mass was poorly optimised
A sampling protocol at a mine was implemented to have a residual uncertainty no more than ±10 % relative for the gold content estimate generated by industry standard 30-g fire assay. A thorough investigation of the necessary sample mass to assay to reach a 10 % relative uncertainty revealed that the necessary sample mass to assay using cyanide bottle roll or gravity concentration was at least 3000 g.
Therefore, the presently used protocol was using assay samples two orders of magnitude too small. The resulting so-called huge uncertainty was definitely a flagrant error, however, due to the fact that the people in charge of the project failed to optimise their sampling protocol because of their total ignorance of the TOS.
In this case the use of the word uncertainty would be totally inappropriate and highly misleading; it is very clear that a huge mistake had been made.
Case #2: A non-probabilistic, therefore, incorrect sampling device was used
The content of a copper concentrate shipment was sampled at the receiving port. The copper concentrate was unloaded onto a conveyor belt. Every five minutes an operator using a scoop would collect an increment at the discharge of the belt. The composite sample was sent to the laboratory for assaying copper.
A QA/QC programme collecting interleaved increments to assess the uncertainty affecting each copper assay was implemented. Because the scoop used by the operator was not an equi-probabilistic sampling device and consisted of an operator-dependent grab sampling practice, the use of the word uncertainty would be totally inappropriate and highly misleading. It is very clear that a huge mistake had been made using an increment sampling device transgressing the most elementary rules of sampling correctness. The word error would be the only appropriate word to use because of the ignorance of the management team involved.
Case #3: A well-optimised sampling protocol generated an uncertainly well within well-defined Data Quality Objectives
A manager asked the laboratory to assay the calcium content of a cement clinker within a well-defined Data Quality Objective, set at ±3 % relative. The sampling and subsampling devices were fully in compliance with the TOS to prevent the occurrence of a sampling bias due to increment delimitation errors (IDE) and increment extraction errors (IEE).
The QA/QC programme revealed that the residual uncertainty affecting the assaying of calcium was only ±2 % relative.
In this case it is correct and totally appropriate to use the word uncertainty because the management team was competent at performing a qualified sampling job.
A statistical aside
As an interesting aside, some (other) statisticians in fact have no problem using the term “error”. The eminent statistician-educator David S. Salsburg has produced a wonderful popularising book: Errors, Blunders and Lies – How to tell the Difference,5 in which there is no problem using the term error and no hesitating in finger-pointing regarding how and how measurement or observation uncertainties arise. Such will always manifest themselves when observations or measurements are repeated is the message. But one is obliged to do something about this situation, and this is where statistics arrives as a knight on a white horse—enter classical statistics. It is worth noting that this type of error is solely related to measurement/observation, the only uncertainty-generating source in this realm. In the present TOS context, this type of error is identical to the analytical error, TAE. However, there is here no recognition or acknowledgement of the situation in which a much more complex process is needed before one can perform the act of “observation/measurement”, i.e. analysis. This process is, of course, the complete lot-to-aliquot sampling process, which has the, for many, unknown characteristic that the process itself will influence the outcome of analysis; the sampling process itself will incur error effects if not in compliance with the TOS. Thus, another sampling procedure, another selection of equipment, another sampler (if not TOS-competent) at work, will give rise to a principally different analytical result. This is a “measurement error” of a fundamentally different nature that what is conceived of in statistics.
Thus, the TOS community is justified in establishing the error vs uncertainty context promulgated above: repeated sampling-plus-analysis is taking place within the full TSE + TAE framework and its attending consequences, and someone (a legal person) or something (standard, guide, norm-giving document etc. which are also legal persons) is responsible for dealing with the effects due to heterogeneity in a rational fashion. Sampling errors give rise to varying uncertainties in the analytical data base, many of which can be dealt with very effectively, however (reduced, CSE), but some of which are fatal: professional samplers are always obliged to rid of all those pesky ISE!
The TOS vs MU
Esbensen and Wagner treated the complicated relationship between the TOS and the concept of Measurement Uncertainty (MU) in all pertinent details: see their concise summary:6
“A critical assessment of GUM and the EURACHEM guide shows that not all influential uncertainty sources are considered with respect to their full MU impacts. In particular, effects caused by ISEs are insufficiently defined and integrated. While GUM exclusively focuses on estimating the analytical MU, the EURACHEM guide indicates and incorporates some error sources related to sampling (mainly only the Fundamental Sampling Error), but detailed analysis reveal several deficiencies compared to TOS’ full sampling-error framework. While the EURACHEM guide acknowledges the existence of the CSEs, it stays with the assumption that all other sampling error sources have been eliminated by other parties—which gives no practical help to the sampler/analyst relying on MU alone.”
“By excluding both the concept of, and the risk incurred by, the inconstant sampling bias, the sampler/analyst may well not even beware of the risk that the effective MU estimate will be principally different each time it is re-estimated. The user is left without the crucial understanding that ISE effects unavoidably result in uncontrolled and unquantifiable, inflated MUtotal estimates, i.e. the sampling variance, the sampling uncertainty, is increased because of incorrect sampling errors. Only the TOS offers complete theoretical and practical understanding of all features related to heterogeneity and full practical insight into the intricacies of the sampling process when confronting the gamut of heterogeneity manifestations. Closing this gap between TOS and MU necessitates a certain minimum TOS competence, and practical confidence, that all sampling processes can indeed be correct (bias-free sampling), opening up for representative, or fit-for-purpose representative sampling, which is the only way to an acceptable level of uncertainty. This minimum competency is outlined for example in the standard, DS 3077.”7
“To derive a valid estimate of the complete uncertainty for any measurement procedure (sampling-and-analysis), all ISEs and CSEs, as well as the TAE (MUanalysis) must be considered in their proper place. This opens the way to a unified sampling-and-analysis responsibility. A detailed analysis of MU points out that TOS can simply be inducted as an essential first part in the complete measurement-process framework, taking charge and responsibility of all sampling issues at all scales along the entire lot-to-aliquot process. What is called for is a constructive integration between TOS and MU, allowing reconciliation of these two frameworks that all too long have been considered only antagonistically.”
(© Elsevier 2014. Reprinted from Reference 6 (https://doi.org/10.1016/j.trac.2014.02.007), with permission from Elsevier)
Conclusion
The use of the term uncertainty should apply only in cases where the legal person responsible for sampling (management) has successfully trained its staff appropriately (scientists, technicians, front line samplers) and has taken a solemn commitment to apply the principles and recommendations offered by the TOS. Anything less will be an irresponsible, fragrant error. All sampling must be fully accountable.
The evergreen confusion arising from lack of distinction, or from synonymous usage of the terms error vs uncertainty, is unfortunately often also broadened by a prominent lack of proper understanding of the meaning of error vs uncertainty vs MU.
References
- M.H. Bundy, Uncertainty In Science, Statistics. Encyclopedia.com [accessed 11 August 2022]. https://www.encyclopedia.com/environment/encyclopedias-almanacs-transcripts-and-maps/uncertainty-science-statistics
- P.M. Gy, “L’Echantillonage de minerais en vrac” (Sampling of particulate materials), Vol. 1. Revue de l’Industrie Minerale 1, Numero Special (Special Issue), 15 January (1967).
- F.F. Pitard, Pierre Gy’s Theory of Sampling and C.O. Ingamell’s Poisson Process Approach – Pathways to Representative Sampling and Appropriate Industrial Standards. Doctoral thesis, Aalborg University, Campus Esbjerg (2009). ISBN: 978-87-7606-032-9
- F.F. Pitard, Theory of Sampling and Sampling Practice, 3rd Edn. CRC Press, Boca Raton, Florida (2019). https://doi.org/10.1201/9781351105934
- D.S. Salsburg, Errors, Blunders and Lies – How to tell the Difference. CRC Press, Taylor & Francis Group, Baton Rouge, FL, USA (2017). https://doi.org/10.1201/9781315379081
The book by Salsburg is written in a humorous and very easy-to-read fashion, and also contains an extremely user-friendly introduction to the normal and the Poission distributions and to their wide application fields, both of which are the only statistical tools used in the TOS. Salsburg’s book is greatly recommended for the novice reading these lines … - K.H. Esbensen and C. Wagner, “Theory of Sampling (TOS) versus measurement uncertainty (MU) – a call for integration”, Trends Anal. Chem. (TrAC) 57, 93–106 (2014). https://doi.org/10.1016/j.trac.2014.02.007
- DS 3077. Representative Sampling—Horizontal Standard. Danish Standards (2013). http://www.ds.dk
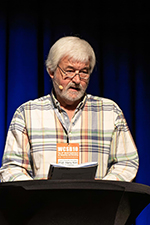
Kim Esbensen
Kim H. Esbensen, PhD, Dr (hon), has been research professor in Geoscience Data Analysis and Sampling at GEUS, the National Geological Surveys of Denmark and Greenland (2010–2015), chemometrics & sampling professor at Aalborg University, Denmark (2001–2015), professor (Process Analytical Technologies) at Telemark Institute of Technology, Norway (1990–2000 and 2010–2015) and professeur associé, Université du Québec à Chicoutimi (2013–2016). From 2015 he phased out a more than 30-year academic career for a new quest as an independent researcher and consultant. But as he could not terminate his love for teaching, he is still very active as an international visiting, guest and affiliate professor. A geologist/geochemist/metallurgist/data analyst of training, he has been working 20+ years in the forefront of chemometrics, but since 2000 has devoted most of his scientific R&D to the theme of representative sampling of heterogeneous materials, processes and systems: Theory of Sampling (TOS), PAT (Process Analytical Technology) and chemometrics. He is a member of several scientific societies and has published over 250 peer-reviewed papers and is the author of a widely used textbook in Multivariate Data Analysis (35,000 copies), which was published in its 6th edition in 2018. He was chairman of the taskforce behind the world’s first horizontal (matrix-independent) sampling standard DS 3077 (2013). He is editor of the science magazine TOS forum and this Sampling Column. In 2020 he published the textbook: Introduction to the Theory and Practice of Sampling (impopen.com/sampling). Esbensen was awarded the prestigious Pierre Gy’s Gold Medal for excellence in promoting and teaching the Theory of Sampling in 2013 (WCSB6).
[email protected]
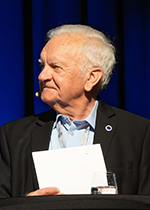
Francis Pitard
Dr Francis F. Pitard is a consulting expert in Sampling, Statistical Process Control (SPC) and Total Quality Management (TQM). He is President of Francis Pitard Sampling Consultants in Broomfield, Colorado, USA. Dr Pitard has six years of experience with the French Atomic Energy Commission and fifteen years with Amax Extractive R&D. He teaches Sampling Theory for the Continuing Education Offices of the Colorado School of Mines. He has a Doctorate in Technologies from Aalborg University in Denmark. He is the author of Theory of Sampling and Sampling Practice (Third Edition 2019). He is the recipient of the prestigious Pierre Gy’s Gold Medal for excellence in promoting and teaching the Theory of Sampling.
[email protected]